Ever dreamt of transforming your online store into a conversion machine, churning out sales like clockwork? A/B testing is a powerful tool in the digital marketer’s arsenal, crucial for making data-driven decisions. By comparing two versions of a webpage or app, A/B testing reveals which version performs better based on statistical significance, confidence, and power. But navigating the world of statistics within A/B testing can feel like deciphering hieroglyphics. Fear not, fellow e-commerce warriors! This article cuts through the jargon and equips you with the knowledge to unlock the power of statistical significance, confidence intervals, and power. We’ll show you how to interpret these concepts for A/B testing success, so you can confidently make data-driven decisions and watch your sales soar.
This article dives into the world of A/B testing, specifically focusing on statistical significance, confidence intervals, and power. We’ll break down these terms in a way that makes them relatable, even for those who might have sworn off maths forever!
Why Do We Need Statistics in A/B Testing?
Imagine you’re running an online store. You’ve designed a snazzy new product page, convinced it’ll skyrocket sales. But how do you know it’s actually better than the old one? Here’s where A/B testing comes in. It lets you compare two versions of something (like your product pages) and see which one performs better.
However, A/B testing results can be fickle. Sometimes, a version might seem to win just by chance. That’s where statistics come in – they help us understand the likelihood of our results being real and not just a random blip.
Statistical Significance: Separating Fact from Fluke
Let’s say you run an A/B test on your product page headline. The new headline seems to generate a 5% increase in clicks. But is this a true reflection of its effectiveness, or could it simply be a random fluctuation?
Statistical significance helps us answer that. It tells us the probability that the observed difference (5% increase) isn’t due to chance. Typically, we aim for a significance level of 95%. This means if we ran the test 100 times with the same setup, we’d expect to see a 5% increase (or something similar) 95 times out of 100, suggesting a genuine impact.
Here’s a fun analogy: imagine flipping a coin. If it lands on heads five times in a row, you might think it’s a lucky coin. But statistically, this can happen by chance. Statistical significance helps us determine if the observed outcome (five heads) is more likely due to chance (like the coin) or a real underlying difference (like your new product page headline).
Confidence Intervals: Embracing the Range of Reality
So, statistical significance tells us there’s probably a real difference, but how big is that difference likely to be? Confidence intervals come to the rescue!
Think of them as a range of values where the true effect (like the actual increase in clicks) is likely to lie. It’s like estimating how much you’ll spend on groceries – you might say, “I’ll probably need between £20 and £30.” Confidence intervals work similarly, giving us a sense of how much the results might vary depending on factors like sample size or random chance.
For example, your A/B test might show a 5% increase in clicks with a 95% confidence interval of 2% to 8%. This means we’re 95% confident that the true increase in clicks falls somewhere between 2% and 8%.
Power Up Your A/B Tests: The Importance of Sample Size
Imagine you have a weak radar – it might miss a faint signal from a distant ship. Similarly, low statistical power in A/B testing can lead us to miss real differences between your new product page and the old one.
Power refers to the test’s ability to detect a true effect if one exists. It depends on three key factors:
- Sample Size: The more people exposed to each version of your product page (the sample size), the higher the power.
- Effect Size: The bigger the actual difference between your new page and the old one (the effect size), the easier it is to detect.
- Significance Level: The stricter your significance level (like aiming for 95% confidence), the lower the power (but the more reliable the results).
There’s a balancing act here. Ideally, you want a good sample size, a decent effect size you’re looking for (e.g., a 10% increase in clicks), and a reasonable significance level (like 95%). A/B testing tools often have calculators to help you determine the optimal sample size for your desired power.
The Takeaway: Statistics are Your A/B Testing Superpower
Statistical significance, confidence intervals, and power might sound intimidating, but they’re your allies in the world of A/B testing. By understanding them, you can:
- Be confident in your results: Statistical significance helps you avoid mistaking random fluctuations for real effects.
- Estimate the range of possibilities: Confidence intervals give you a sense of how much the results might vary.
- Maximize your chances of detecting real differences: Power ensures you don’t miss out on valuable insights due to a weak test design.
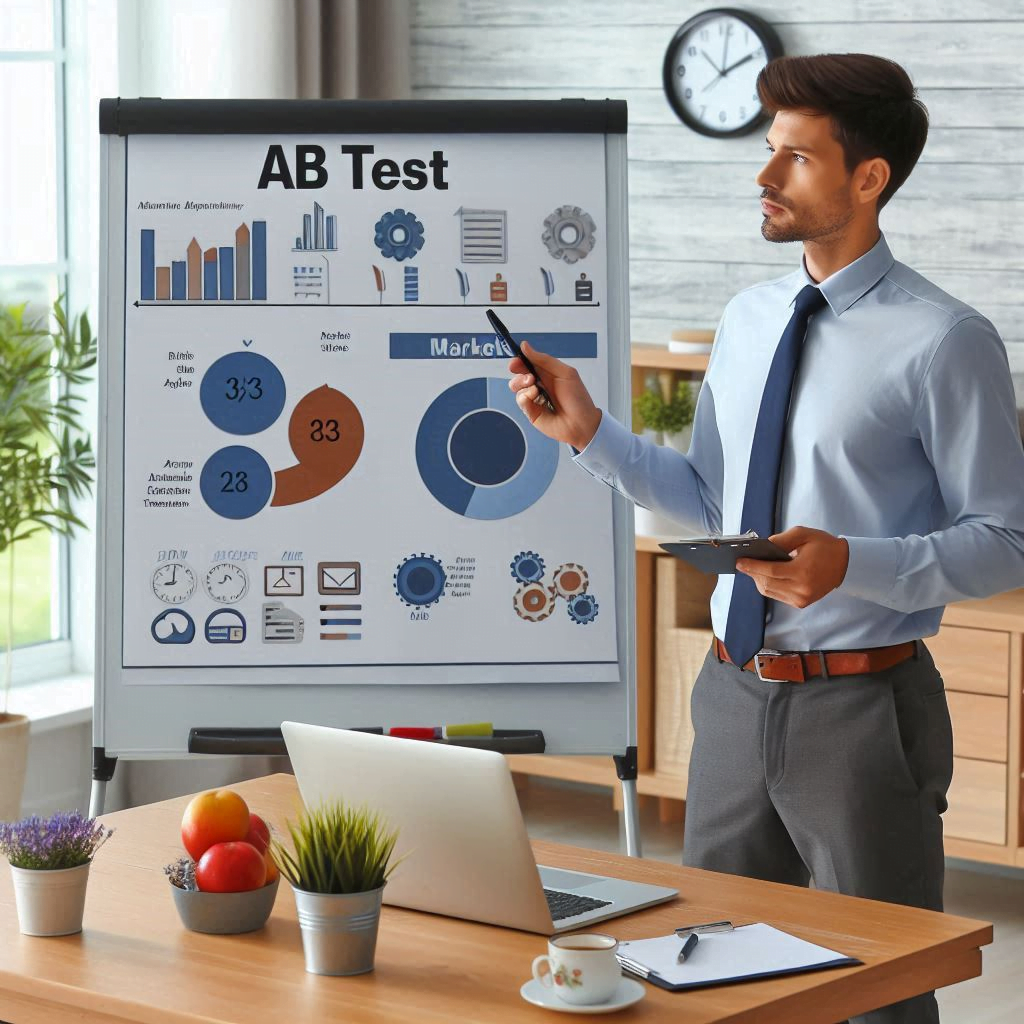
A/B Testing Like a Pro: Putting Statistics into Action
Now that we’ve demystified statistical significance, confidence intervals, and power, let’s see how they translate into real-world A/B testing scenarios.
Example 1: Optimising Your Call to Action Button
You’re not sure if your current “Buy Now” button is converting as well as it could. You design a new button with a different colour and text (“Add to Cart”). You run an A/B test, and after gathering enough data, you get the following results:
- Statistical Significance: 92% (not quite reaching the golden standard of 95%)
- Confidence Interval: Increase in conversions of 3% to 8%
Interpretation: There’s a good chance the new button is performing better, but the significance level is a bit lower than ideal. The confidence interval tells us the true increase in conversions could be anywhere between 3% and 8%.
Action: Consider extending the test duration to increase sample size and potentially push the significance level closer to 95%.
Example 2: Revamping Your Product Description
You suspect your current product description might be too long and turn off potential customers. You create a shorter, punchier version and run an A/B test. Here’s what you see:
- Statistical Significance: 98% (strong indication of a real difference)
- Confidence Interval: Increase in click-through rate of 5% to 12%
Interpretation: The new description is likely a winner! The high significance level suggests a genuine effect, and the confidence interval shows a substantial potential increase in click-through rates.
Action: Implement the new product description across your website.
Remember:
- A/B testing is an iterative process. Don’t get discouraged if your first test doesn’t yield earth-shattering results. Keep testing and refining based on the data you collect.
- Statistical concepts like significance and power might seem complex at first, but with practice, they become second nature. There are plenty of online resources and A/B testing tools that offer guidance and calculators to help you design statistically sound experiments.
By embracing the power of statistics, you can transform A/B testing from a guessing game into a data-driven approach to eCommerce success. So, go forth, conquer those product pages, and optimise your way to a thriving online store!